Function score queryedit
The function_score
allows you to modify the score of documents that are
retrieved by a query. This can be useful if, for example, a score
function is computationally expensive and it is sufficient to compute
the score on a filtered set of documents.
To use function_score
, the user has to define a query and one or
more functions, that compute a new score for each document returned
by the query.
function_score
can be used with only one function like this:
GET /_search { "query": { "function_score": { "query": { "match_all": {} }, "boost": "5", "random_score": {}, "boost_mode":"multiply" } } }
See Function score for a list of supported functions. |
Furthermore, several functions can be combined. In this case one can optionally choose to apply the function only if a document matches a given filtering query
GET /_search { "query": { "function_score": { "query": { "match_all": {} }, "boost": "5", "functions": [ { "filter": { "match": { "test": "bar" } }, "random_score": {}, "weight": 23 }, { "filter": { "match": { "test": "cat" } }, "weight": 42 } ], "max_boost": 42, "score_mode": "max", "boost_mode": "multiply", "min_score" : 42 } } }
Boost for the whole query. |
|
See Function score for a list of supported functions. |
The scores produced by the filtering query of each function do not matter.
If no filter is given with a function this is equivalent to specifying
"match_all": {}
First, each document is scored by the defined functions. The parameter
score_mode
specifies how the computed scores are combined:
|
scores are multiplied (default) |
|
scores are summed |
|
scores are averaged |
|
the first function that has a matching filter is applied |
|
maximum score is used |
|
minimum score is used |
Because scores can be on different scales (for example, between 0 and 1 for decay functions but arbitrary for field_value_factor
) and also
because sometimes a different impact of functions on the score is desirable, the score of each function can be adjusted with a user defined
weight
. The weight
can be defined per function in the functions
array (example above) and is multiplied with the score computed by
the respective function.
If weight is given without any other function declaration, weight
acts as a function that simply returns the weight
.
In case score_mode
is set to avg
the individual scores will be combined by a weighted average.
For example, if two functions return score 1 and 2 and their respective weights are 3 and 4, then their scores will be combined as
(1*3+2*4)/(3+4)
and not (1*3+2*4)/2
.
The new score can be restricted to not exceed a certain limit by setting
the max_boost
parameter. The default for max_boost
is FLT_MAX.
The newly computed score is combined with the score of the
query. The parameter boost_mode
defines how:
|
query score and function score is multiplied (default) |
|
only function score is used, the query score is ignored |
|
query score and function score are added |
|
average |
|
max of query score and function score |
|
min of query score and function score |
By default, modifying the score does not change which documents match. To exclude
documents that do not meet a certain score threshold the min_score
parameter can be set to the desired score threshold.
For min_score
to work, all documents returned by the query need to be scored and then filtered out one by one.
The function_score
query provides several types of score functions.
-
script_score
-
weight
-
random_score
-
field_value_factor
-
decay functions:
gauss
,linear
,exp
Script scoreedit
The script_score
function allows you to wrap another query and customize
the scoring of it optionally with a computation derived from other numeric
field values in the doc using a script expression. Here is a
simple sample:
GET /_search { "query": { "function_score": { "query": { "match": { "message": "elasticsearch" } }, "script_score" : { "script" : { "source": "Math.log(2 + doc['likes'].value)" } } } } }
In Elasticsearch, all document scores are positive 32-bit floating point numbers.
If the script_score
function produces a score with greater precision, it is
converted to the nearest 32-bit float.
Similarly, scores must be non-negative. Otherwise, Elasticsearch returns an error.
On top of the different scripting field values and expression, the
_score
script parameter can be used to retrieve the score based on the
wrapped query.
Scripts compilation is cached for faster execution. If the script has parameters that it needs to take into account, it is preferable to reuse the same script, and provide parameters to it:
GET /_search { "query": { "function_score": { "query": { "match": { "message": "elasticsearch" } }, "script_score" : { "script" : { "params": { "a": 5, "b": 1.2 }, "source": "params.a / Math.pow(params.b, doc['likes'].value)" } } } } }
Note that unlike the custom_score
query, the
score of the query is multiplied with the result of the script scoring. If
you wish to inhibit this, set "boost_mode": "replace"
Weightedit
The weight
score allows you to multiply the score by the provided
weight
. This can sometimes be desired since boost value set on
specific queries gets normalized, while for this score function it does
not. The number value is of type float.
"weight" : number
Randomedit
The random_score
generates scores that are uniformly distributed from 0 up to
but not including 1. By default, it uses the internal Lucene doc ids as a
source of randomness, which is very efficient but unfortunately not
reproducible since documents might be renumbered by merges.
In case you want scores to be reproducible, it is possible to provide a seed
and field
. The final score will then be computed based on this seed, the
minimum value of field
for the considered document and a salt that is computed
based on the index name and shard id so that documents that have the same
value but are stored in different indexes get different scores. Note that
documents that are within the same shard and have the same value for field
will however get the same score, so it is usually desirable to use a field that
has unique values for all documents. A good default choice might be to use the
_seq_no
field, whose only drawback is that scores will change if the document
is updated since update operations also update the value of the _seq_no
field.
It was possible to set a seed without setting a field, but this has been
deprecated as this requires loading fielddata on the _id
field which consumes
a lot of memory.
GET /_search { "query": { "function_score": { "random_score": { "seed": 10, "field": "_seq_no" } } } }
Field Value factoredit
The field_value_factor
function allows you to use a field from a document to
influence the score. It’s similar to using the script_score
function, however,
it avoids the overhead of scripting. If used on a multi-valued field, only the
first value of the field is used in calculations.
As an example, imagine you have a document indexed with a numeric likes
field and wish to influence the score of a document with this field, an example
doing so would look like:
GET /_search { "query": { "function_score": { "field_value_factor": { "field": "likes", "factor": 1.2, "modifier": "sqrt", "missing": 1 } } } }
Which will translate into the following formula for scoring:
sqrt(1.2 * doc['likes'].value)
There are a number of options for the field_value_factor
function:
|
Field to be extracted from the document. |
|
Optional factor to multiply the field value with, defaults to |
|
Modifier to apply to the field value, can be one of: |
Modifier | Meaning |
---|---|
|
Do not apply any multiplier to the field value |
|
Take the common logarithm of the field value.
Because this function will return a negative value and cause an error if used on values
between 0 and 1, it is recommended to use |
|
Add 1 to the field value and take the common logarithm |
|
Add 2 to the field value and take the common logarithm |
|
Take the natural logarithm of the field value.
Because this function will return a negative value and cause an error if used on values
between 0 and 1, it is recommended to use |
|
Add 1 to the field value and take the natural logarithm |
|
Add 2 to the field value and take the natural logarithm |
|
Square the field value (multiply it by itself) |
|
Take the square root of the field value |
|
Reciprocate the field value, same as |
-
missing
- Value used if the document doesn’t have that field. The modifier and factor are still applied to it as though it were read from the document.
Scores produced by the field_value_score
function must be
non-negative, otherwise an error will be thrown. The log
and ln
modifiers
will produce negative values if used on values between 0 and 1. Be sure to limit
the values of the field with a range filter to avoid this, or use log1p
and
ln1p
.
Keep in mind that taking the log() of 0, or the square root of a
negative number is an illegal operation, and an exception will be thrown. Be
sure to limit the values of the field with a range filter to avoid this, or use
log1p
and ln1p
.
Decay functionsedit
Decay functions score a document with a function that decays depending on the distance of a numeric field value of the document from a user given origin. This is similar to a range query, but with smooth edges instead of boxes.
To use distance scoring on a query that has numerical fields, the user
has to define an origin
and a scale
for each field. The origin
is needed to define the “central point” from which the distance
is calculated, and the scale
to define the rate of decay. The
decay function is specified as
"DECAY_FUNCTION": { "FIELD_NAME": { "origin": "11, 12", "scale": "2km", "offset": "0km", "decay": 0.33 } }
The |
|
The specified field must be a numeric, date, or geo-point field. |
In the above example, the field is a geo_point
and origin can
be provided in geo format. scale
and offset
must be given with a unit in
this case. If your field is a date field, you can set scale
and offset
as
days, weeks, and so on. Example:
GET /_search { "query": { "function_score": { "gauss": { "date": { "origin": "2013-09-17", "scale": "10d", "offset": "5d", "decay" : 0.5 } } } } }
The date format of the origin depends on the |
|
The |
|
The point of origin used for calculating distance. Must be given as a
number for numeric field, date for date fields and geo point for geo fields.
Required for geo and numeric field. For date fields the default is |
|
Required for all types. Defines the distance from origin + offset at which the computed
score will equal |
|
If an |
|
The |
In the first example, your documents might represents hotels and contain a geo location field. You want to compute a decay function depending on how far the hotel is from a given location. You might not immediately see what scale to choose for the gauss function, but you can say something like: "At a distance of 2km from the desired location, the score should be reduced to one third." The parameter "scale" will then be adjusted automatically to assure that the score function computes a score of 0.33 for hotels that are 2km away from the desired location.
In the second example, documents with a field value between 2013-09-12 and 2013-09-22 would get a weight of 1.0 and documents which are 15 days from that date a weight of 0.5.
Supported decay functionsedit
The DECAY_FUNCTION
determines the shape of the decay:
-
gauss
-
Normal decay, computed as:
where
is computed to assure that the score takes the value
decay
at distancescale
fromorigin
+-offset
See Normal decay, keyword
gauss
for graphs demonstrating the curve generated by thegauss
function. -
exp
-
Exponential decay, computed as:
where again the parameter
is computed to assure that the score takes the value
decay
at distancescale
fromorigin
+-offset
See Exponential decay, keyword
exp
for graphs demonstrating the curve generated by theexp
function. -
linear
-
Linear decay, computed as:
.
where again the parameter
s
is computed to assure that the score takes the valuedecay
at distancescale
fromorigin
+-offset
In contrast to the normal and exponential decay, this function actually sets the score to 0 if the field value exceeds twice the user given scale value.
For single functions the three decay functions together with their parameters can be visualized like this (the field in this example called "age"):
Multi-values fieldsedit
If a field used for computing the decay contains multiple values, per default the value closest to the origin is chosen for determining the distance.
This can be changed by setting multi_value_mode
.
|
Distance is the minimum distance |
|
Distance is the maximum distance |
|
Distance is the average distance |
|
Distance is the sum of all distances |
Example:
"DECAY_FUNCTION": { "FIELD_NAME": { "origin": ..., "scale": ... }, "multi_value_mode": "avg" }
Detailed exampleedit
Suppose you are searching for a hotel in a certain town. Your budget is limited. Also, you would like the hotel to be close to the town center, so the farther the hotel is from the desired location the less likely you are to check in.
You would like the query results that match your criterion (for example, "hotel, Nancy, non-smoker") to be scored with respect to distance to the town center and also the price.
Intuitively, you would like to define the town center as the origin and
maybe you are willing to walk 2km to the town center from the hotel.
In this case your origin for the location field is the town center
and the scale is ~2km.
If your budget is low, you would probably prefer something cheap above something expensive. For the price field, the origin would be 0 Euros and the scale depends on how much you are willing to pay, for example 20 Euros.
In this example, the fields might be called "price" for the price of the hotel and "location" for the coordinates of this hotel.
The function for price
in this case would be
and for location
:
Suppose you want to multiply these two functions on the original score, the request would look like this:
GET /_search { "query": { "function_score": { "functions": [ { "gauss": { "price": { "origin": "0", "scale": "20" } } }, { "gauss": { "location": { "origin": "11, 12", "scale": "2km" } } } ], "query": { "match": { "properties": "balcony" } }, "score_mode": "multiply" } } }
Next, we show how the computed score looks like for each of the three possible decay functions.
Normal decay, keyword gauss
edit
When choosing gauss
as the decay function in the above example, the
contour and surface plot of the multiplier looks like this:


Suppose your original search results matches three hotels :
- "Backback Nap"
- "Drink n Drive"
- "BnB Bellevue".
"Drink n Drive" is pretty far from your defined location (nearly 2 km) and is not too cheap (about 13 Euros) so it gets a low factor a factor of 0.56. "BnB Bellevue" and "Backback Nap" are both pretty close to the defined location but "BnB Bellevue" is cheaper, so it gets a multiplier of 0.86 whereas "Backpack Nap" gets a value of 0.66.
Exponential decay, keyword exp
edit
When choosing exp
as the decay function in the above example, the
contour and surface plot of the multiplier looks like this:


Linear decay, keyword linear
edit
When choosing linear
as the decay function in the above example, the
contour and surface plot of the multiplier looks like this:
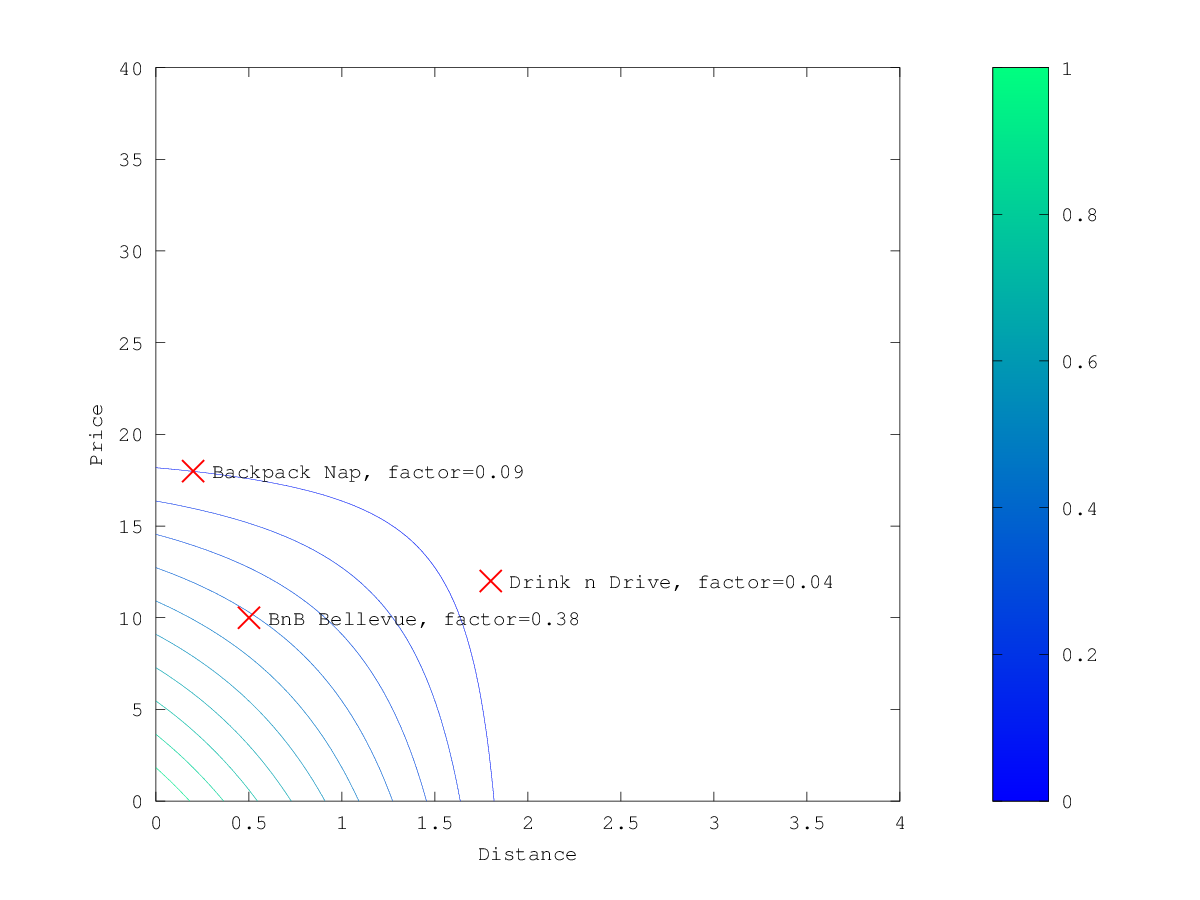
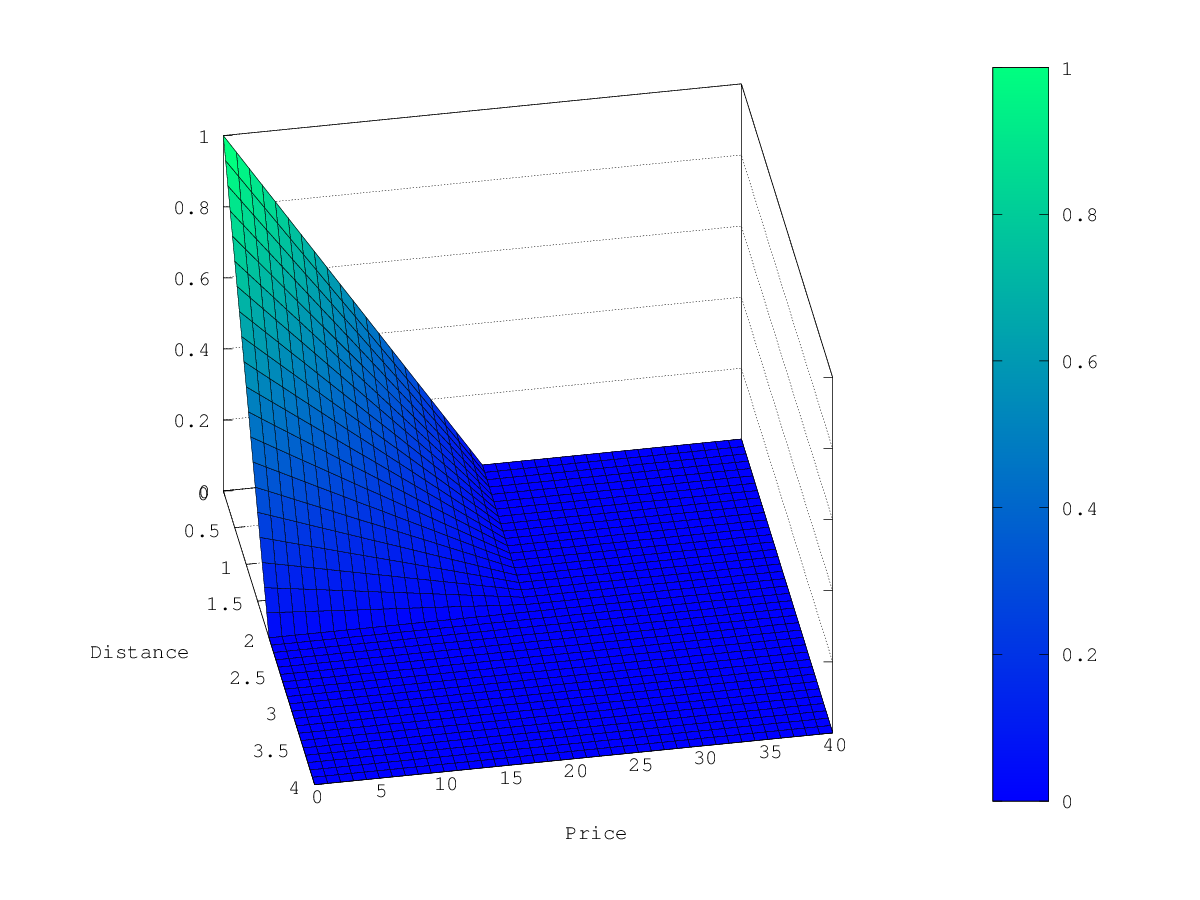
Supported fields for decay functionsedit
Only numeric, date, and geo-point fields are supported.
What if a field is missing?edit
If the numeric field is missing in the document, the function will return 1.
- Elasticsearch Guide: other versions:
- What is Elasticsearch?
- What’s new in 7.7
- Getting started with Elasticsearch
- Set up Elasticsearch
- Installing Elasticsearch
- Configuring Elasticsearch
- Setting JVM options
- Secure settings
- Auditing settings
- Circuit breaker settings
- Cluster-level shard allocation and routing settings
- Cross-cluster replication settings
- Discovery and cluster formation settings
- Field data cache settings
- HTTP
- Index lifecycle management settings
- Index recovery settings
- Indexing buffer settings
- License settings
- Local gateway settings
- Logging configuration
- Machine learning settings
- Monitoring settings
- Node
- Network settings
- Node query cache settings
- Search settings
- Security settings
- Shard request cache settings
- Snapshot lifecycle management settings
- SQL access settings
- Transforms settings
- Transport
- Thread pools
- Watcher settings
- Important Elasticsearch configuration
- Important System Configuration
- Bootstrap Checks
- Heap size check
- File descriptor check
- Memory lock check
- Maximum number of threads check
- Max file size check
- Maximum size virtual memory check
- Maximum map count check
- Client JVM check
- Use serial collector check
- System call filter check
- OnError and OnOutOfMemoryError checks
- Early-access check
- G1GC check
- All permission check
- Discovery configuration check
- Bootstrap Checks for X-Pack
- Starting Elasticsearch
- Stopping Elasticsearch
- Discovery and cluster formation
- Add and remove nodes in your cluster
- Full-cluster restart and rolling restart
- Remote clusters
- Set up X-Pack
- Configuring X-Pack Java Clients
- Plugins
- Upgrade Elasticsearch
- Search your data
- Query DSL
- SQL access
- Overview
- Getting Started with SQL
- Conventions and Terminology
- Security
- SQL REST API
- SQL Translate API
- SQL CLI
- SQL JDBC
- SQL ODBC
- SQL Client Applications
- SQL Language
- Functions and Operators
- Comparison Operators
- Logical Operators
- Math Operators
- Cast Operators
- LIKE and RLIKE Operators
- Aggregate Functions
- Grouping Functions
- Date/Time and Interval Functions and Operators
- Full-Text Search Functions
- Mathematical Functions
- String Functions
- Type Conversion Functions
- Geo Functions
- Conditional Functions And Expressions
- System Functions
- Reserved keywords
- SQL Limitations
- Aggregations
- Metrics Aggregations
- Avg Aggregation
- Weighted Avg Aggregation
- Boxplot Aggregation
- Cardinality Aggregation
- Stats Aggregation
- Extended Stats Aggregation
- Geo Bounds Aggregation
- Geo Centroid Aggregation
- Max Aggregation
- Min Aggregation
- Median Absolute Deviation Aggregation
- Percentiles Aggregation
- Percentile Ranks Aggregation
- Scripted Metric Aggregation
- String Stats Aggregation
- Sum Aggregation
- Top Hits Aggregation
- Top Metrics Aggregation
- Value Count Aggregation
- Bucket Aggregations
- Adjacency Matrix Aggregation
- Auto-interval Date Histogram Aggregation
- Children Aggregation
- Composite aggregation
- Date histogram aggregation
- Date Range Aggregation
- Diversified Sampler Aggregation
- Filter Aggregation
- Filters Aggregation
- Geo Distance Aggregation
- GeoHash grid Aggregation
- GeoTile Grid Aggregation
- Global Aggregation
- Histogram Aggregation
- IP Range Aggregation
- Missing Aggregation
- Nested Aggregation
- Parent Aggregation
- Range Aggregation
- Rare Terms Aggregation
- Reverse nested Aggregation
- Sampler Aggregation
- Significant Terms Aggregation
- Significant Text Aggregation
- Terms Aggregation
- Subtleties of bucketing range fields
- Pipeline Aggregations
- Bucket Script Aggregation
- Bucket Selector Aggregation
- Bucket Sort Aggregation
- Avg Bucket Aggregation
- Max Bucket Aggregation
- Min Bucket Aggregation
- Sum Bucket Aggregation
- Cumulative Cardinality Aggregation
- Cumulative Sum Aggregation
- Derivative Aggregation
- Percentiles Bucket Aggregation
- Moving Average Aggregation
- Moving Function Aggregation
- Serial Differencing Aggregation
- Stats Bucket Aggregation
- Extended Stats Bucket Aggregation
- Matrix Aggregations
- Caching heavy aggregations
- Returning only aggregation results
- Aggregation Metadata
- Returning the type of the aggregation
- Indexing aggregation results with transforms
- Metrics Aggregations
- Scripting
- Mapping
- Text analysis
- Overview
- Concepts
- Configure text analysis
- Built-in analyzer reference
- Tokenizer reference
- Char Group Tokenizer
- Classic Tokenizer
- Edge n-gram tokenizer
- Keyword Tokenizer
- Letter Tokenizer
- Lowercase Tokenizer
- N-gram tokenizer
- Path Hierarchy Tokenizer
- Path Hierarchy Tokenizer Examples
- Pattern Tokenizer
- Simple Pattern Tokenizer
- Simple Pattern Split Tokenizer
- Standard Tokenizer
- Thai Tokenizer
- UAX URL Email Tokenizer
- Whitespace Tokenizer
- Token filter reference
- Apostrophe
- ASCII folding
- CJK bigram
- CJK width
- Classic
- Common grams
- Conditional
- Decimal digit
- Delimited payload
- Dictionary decompounder
- Edge n-gram
- Elision
- Fingerprint
- Flatten graph
- Hunspell
- Hyphenation decompounder
- Keep types
- Keep words
- Keyword marker
- Keyword repeat
- KStem
- Length
- Limit token count
- Lowercase
- MinHash
- Multiplexer
- N-gram
- Normalization
- Pattern capture
- Pattern replace
- Phonetic
- Porter stem
- Predicate script
- Remove duplicates
- Reverse
- Shingle
- Snowball
- Stemmer
- Stemmer override
- Stop
- Synonym
- Synonym graph
- Trim
- Truncate
- Unique
- Uppercase
- Word delimiter
- Word delimiter graph
- Character filters reference
- Normalizers
- Index modules
- Ingest node
- Pipeline Definition
- Accessing Data in Pipelines
- Conditional Execution in Pipelines
- Handling Failures in Pipelines
- Enrich your data
- Processors
- Append Processor
- Bytes Processor
- Circle Processor
- Convert Processor
- CSV Processor
- Date Processor
- Date Index Name Processor
- Dissect Processor
- Dot Expander Processor
- Drop Processor
- Enrich Processor
- Fail Processor
- Foreach Processor
- GeoIP Processor
- Grok Processor
- Gsub Processor
- HTML Strip Processor
- Inference Processor
- Join Processor
- JSON Processor
- KV Processor
- Lowercase Processor
- Pipeline Processor
- Remove Processor
- Rename Processor
- Script Processor
- Set Processor
- Set Security User Processor
- Split Processor
- Sort Processor
- Trim Processor
- Uppercase Processor
- URL Decode Processor
- User Agent processor
- ILM: Manage the index lifecycle
- Monitor a cluster
- Frozen indices
- Roll up or transform your data
- Set up a cluster for high availability
- Snapshot and restore
- Secure a cluster
- Overview
- Configuring security
- User authentication
- Built-in users
- Internal users
- Token-based authentication services
- Realms
- Realm chains
- Active Directory user authentication
- File-based user authentication
- LDAP user authentication
- Native user authentication
- OpenID Connect authentication
- PKI user authentication
- SAML authentication
- Kerberos authentication
- Integrating with other authentication systems
- Enabling anonymous access
- Controlling the user cache
- Configuring SAML single-sign-on on the Elastic Stack
- Configuring single sign-on to the Elastic Stack using OpenID Connect
- User authorization
- Built-in roles
- Defining roles
- Security privileges
- Document level security
- Field level security
- Granting privileges for indices and aliases
- Mapping users and groups to roles
- Setting up field and document level security
- Submitting requests on behalf of other users
- Configuring authorization delegation
- Customizing roles and authorization
- Enabling audit logging
- Encrypting communications
- Restricting connections with IP filtering
- Cross cluster search, clients, and integrations
- Tutorial: Getting started with security
- Tutorial: Encrypting communications
- Troubleshooting
- Some settings are not returned via the nodes settings API
- Authorization exceptions
- Users command fails due to extra arguments
- Users are frequently locked out of Active Directory
- Certificate verification fails for curl on Mac
- SSLHandshakeException causes connections to fail
- Common SSL/TLS exceptions
- Common Kerberos exceptions
- Common SAML issues
- Internal Server Error in Kibana
- Setup-passwords command fails due to connection failure
- Failures due to relocation of the configuration files
- Limitations
- Alerting on cluster and index events
- Command line tools
- How To
- Glossary of terms
- REST APIs
- API conventions
- cat APIs
- cat aliases
- cat allocation
- cat anomaly detectors
- cat count
- cat data frame analytics
- cat datafeeds
- cat fielddata
- cat health
- cat indices
- cat master
- cat nodeattrs
- cat nodes
- cat pending tasks
- cat plugins
- cat recovery
- cat repositories
- cat shards
- cat segments
- cat snapshots
- cat task management
- cat templates
- cat thread pool
- cat trained model
- cat transforms
- Cluster APIs
- Cross-cluster replication APIs
- Document APIs
- Enrich APIs
- Explore API
- Index APIs
- Add index alias
- Analyze
- Clear cache
- Clone index
- Close index
- Create index
- Delete index
- Delete index alias
- Delete index template
- Flush
- Force merge
- Freeze index
- Get field mapping
- Get index
- Get index alias
- Get index settings
- Get index template
- Get mapping
- Index alias exists
- Index exists
- Index recovery
- Index segments
- Index shard stores
- Index stats
- Index template exists
- Open index
- Put index template
- Put mapping
- Refresh
- Rollover index
- Shrink index
- Split index
- Synced flush
- Type exists
- Unfreeze index
- Update index alias
- Update index settings
- Index lifecycle management API
- Ingest APIs
- Info API
- Licensing APIs
- Machine learning anomaly detection APIs
- Add events to calendar
- Add jobs to calendar
- Close jobs
- Create jobs
- Create calendar
- Create datafeeds
- Create filter
- Delete calendar
- Delete datafeeds
- Delete events from calendar
- Delete filter
- Delete forecast
- Delete jobs
- Delete jobs from calendar
- Delete model snapshots
- Delete expired data
- Estimate model memory
- Find file structure
- Flush jobs
- Forecast jobs
- Get buckets
- Get calendars
- Get categories
- Get datafeeds
- Get datafeed statistics
- Get influencers
- Get jobs
- Get job statistics
- Get machine learning info
- Get model snapshots
- Get overall buckets
- Get scheduled events
- Get filters
- Get records
- Open jobs
- Post data to jobs
- Preview datafeeds
- Revert model snapshots
- Set upgrade mode
- Start datafeeds
- Stop datafeeds
- Update datafeeds
- Update filter
- Update jobs
- Update model snapshots
- Machine learning data frame analytics APIs
- Create data frame analytics jobs
- Create inference trained model
- Delete data frame analytics jobs
- Delete inference trained model
- Evaluate data frame analytics
- Explain data frame analytics API
- Get data frame analytics jobs
- Get data frame analytics jobs stats
- Get inference trained model
- Get inference trained model stats
- Start data frame analytics jobs
- Stop data frame analytics jobs
- Migration APIs
- Reload search analyzers
- Rollup APIs
- Search APIs
- Security APIs
- Authenticate
- Change passwords
- Clear cache
- Clear roles cache
- Create API keys
- Create or update application privileges
- Create or update role mappings
- Create or update roles
- Create or update users
- Delegate PKI authentication
- Delete application privileges
- Delete role mappings
- Delete roles
- Delete users
- Disable users
- Enable users
- Get API key information
- Get application privileges
- Get builtin privileges
- Get role mappings
- Get roles
- Get token
- Get users
- Has privileges
- Invalidate API key
- Invalidate token
- OpenID Connect Prepare Authentication API
- OpenID Connect authenticate API
- OpenID Connect logout API
- SAML prepare authentication API
- SAML authenticate API
- SAML logout API
- SAML invalidate API
- SSL certificate
- Snapshot and restore APIs
- Snapshot lifecycle management API
- Transform APIs
- Usage API
- Watcher APIs
- Definitions
- Breaking changes
- Release notes
- Elasticsearch version 7.7.1
- Elasticsearch version 7.7.0
- Elasticsearch version 7.6.2
- Elasticsearch version 7.6.1
- Elasticsearch version 7.6.0
- Elasticsearch version 7.5.2
- Elasticsearch version 7.5.1
- Elasticsearch version 7.5.0
- Elasticsearch version 7.4.2
- Elasticsearch version 7.4.1
- Elasticsearch version 7.4.0
- Elasticsearch version 7.3.2
- Elasticsearch version 7.3.1
- Elasticsearch version 7.3.0
- Elasticsearch version 7.2.1
- Elasticsearch version 7.2.0
- Elasticsearch version 7.1.1
- Elasticsearch version 7.1.0
- Elasticsearch version 7.0.0
- Elasticsearch version 7.0.0-rc2
- Elasticsearch version 7.0.0-rc1
- Elasticsearch version 7.0.0-beta1
- Elasticsearch version 7.0.0-alpha2
- Elasticsearch version 7.0.0-alpha1